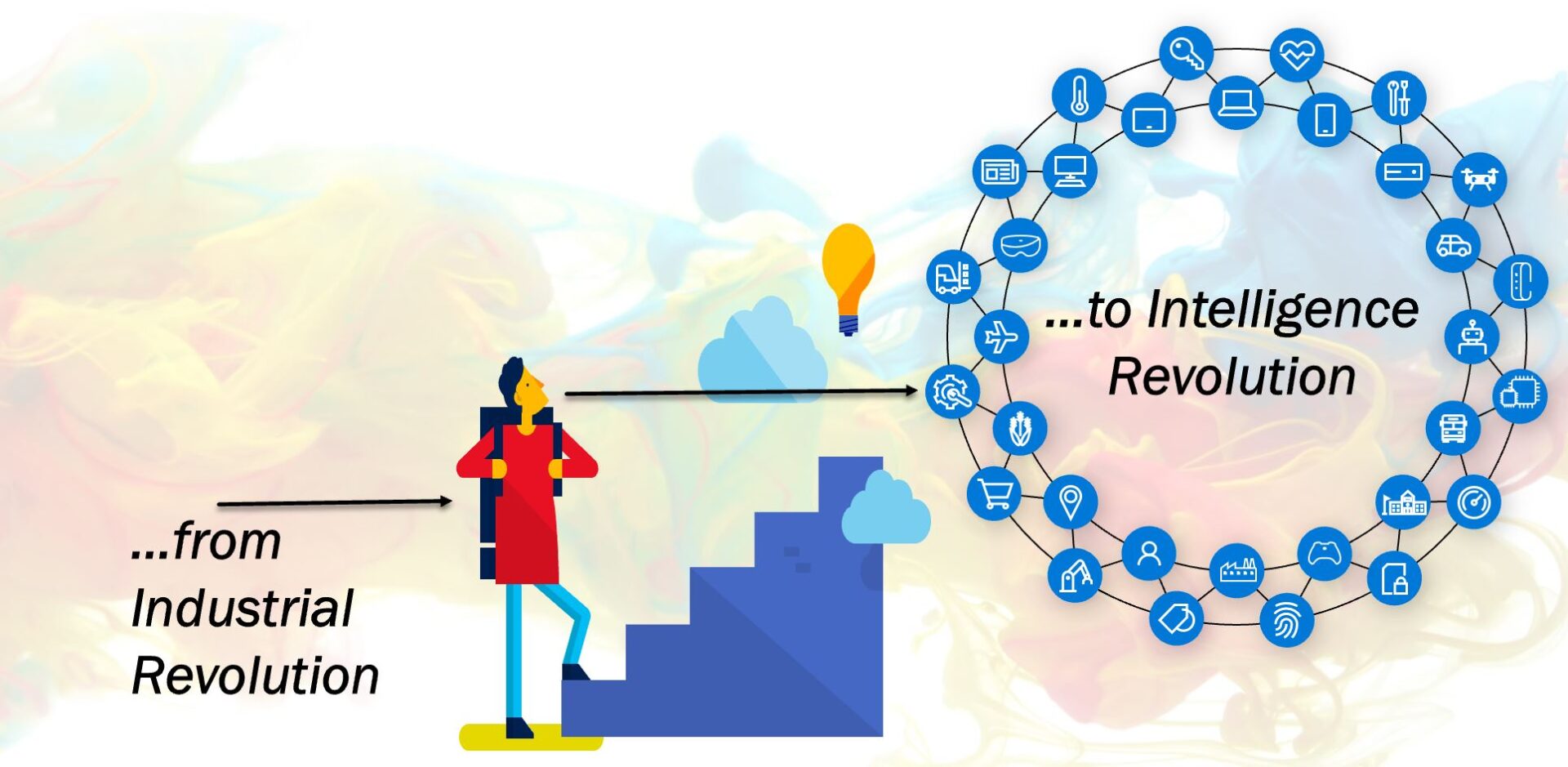
Experiment and measure of business impact is essential for Artificial Intelligence Adoption
Artificial Intelligence is making into our social, enterprise and personal lives faster than what we are expecting. Unlike automation, where handling laundry to assembling cars through well defined rules and speed increase the productivity, AI is different. AI systems take decisions based on historical, live and projected parameters. Key to the transformation from automation to AI is to move from deterministic rule driven outcomes to probabilistic recommendations.
Leading many AI projects from design to production, I realized that traditional approach of build or buy based on product features, cost and customization will not work. In fact this approach in AI applications will result in conflicting expectations on results and lack of clarity on the desired value.
An alternate approach is based on the concept of experimentation and measuring business impact from the initial stages when building AI solution. This will give opportunity for everyone involved to learn the patterns and provide continuous feedback on every iterative cycle of training and testing. This type of reinforcement learning helped to make AI adoption better and remove the fear of failure.
To validate this theory, I, along with my team, analysed AI solutions with key guiding principles:
- First define a simple framework for building intelligence. Intelligence could through vision, voice, documents, sensor data, rule engines etc
- Identify only one domain or scenario to develop AI solution. This will help in controlled scope and good evaluation of the results
- Assume minimal readiness from all parties involved
- Avoid defining the acceptance criteria, but focus on how the outcome from AI in terms of adaptability, cost, productivity and usability
In consultation with teams, we selected different cases like prediction and optimization problems
Here are the key insights, which can be applied in any space on AI adoption:
- The success of AI solution is not based on technology but on the flexibility of solution to learn as per the field trails
- User tolerance of error for an AI driven solution will be very less. Simple gaps in prediction will quickly erode the confidence of using the system
- Don’t wait too long in developing, instead spend more time in the field improving the probabilities and learning from anomalies
- Select just one or two scenarios and drive the value. Never start with enterprise scale AI promise in the beginning which will create fears, confusion and in some cases total revolt
- Time-box the experimentation cycle and evaluate to approve or reject the solution based on business impact. This will help in reducing the cost of failure and moving on with alternative or totally new scenarios